Introduction
Now we have learned about Network Datasets and Network Analysis Layer (NA Layer) in Part 1, how to find routes from one point to another, and among multiple points in Part 2, how to generate service area in Part 3, and how to find closest facility in Part 4, let's move onto the fifth topic - how to create an Origin Destination Cost Matrix
. Please refer to the road map below if you want to revisit the previous topics or jump to the next topic -
- Network Dataset and Network Analysis services (Part 1)
- Find Routes (Part 2)
- Generate Service Area (Part 3)
- Find Closest Facility (Part 4)
- Generate Origin Destination Cost Matrix (You are here!)
- Solve Location Allocation (Part 6)
- Vehicle Routing Problem Service (Part 7)
An origin-destination (OD) cost matrix from multiple origins to multiple destinations, is a table that contains the cost, such as the travel time or travel distance, from each origin to each destination. Additionally, it ranks the destinations that each origin connects to in ascending order based on the minimum cost required to travel from that origin to each destination. When generating an OD cost matrix, you can optionally specify the maximum number of destinations to find for each origin and the maximum time or distance to travel when searching for destinations (1).
The API of Generate Origin Destination Cost Matrix
is useful when you need to find and measure the least-cost paths along the network from multiple origins to multiple destinations, and you can specify the number of destinations to find and a maximum distance to search. Note that, even though the OD cost matrix solver doesn't output lines that follow the network, the values stored in the Lines attribute table reflect the network distance, not the straight-line distance (2).
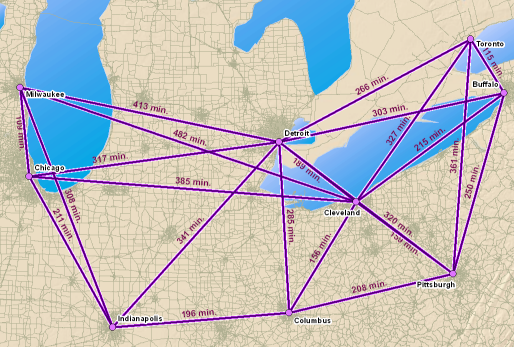
Fig 1. The OD cost matrix found the least-cost paths from each origin to the four nearest destinations. The output shape type was set to produce straight lines (2).
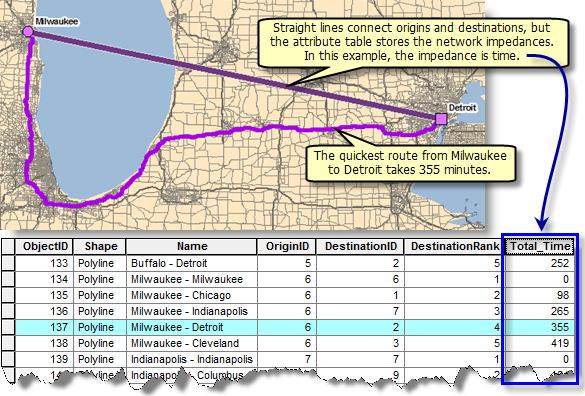
Fig 2. Even though the OD cost matrix solver doesn't output lines that follow the network, the values stored in the Lines attribute table reflect the network distance, not the straight-line distance. This is because by default the displaying option for the output lines in the OD Cost Matrix analysis layer is the generalized straight lines from one point to another. In doing such, the server side can save computation resources and network bandwidth in transferring. However, their cost attributes always report the least-cost network path (3).
This part of guide to Networking Analysis will help you measure the cost of traveling between origins and destinations. When you are ready, let's make your hands dirty with some real implementations!
Differences between Closest Facility and OD cost Matrix
The closest facility and OD cost matrix solvers perform very similar analyses; the main difference, however, is in the output and the computation speed.
OD cost matrix generates results more quickly but cannot return the true shapes of routes or their driving directions. It is designed to quickly solve large M x N problems and, as a result, does not internally contain the information required to generate route shapes and driving directions.
Alternatively, the closest facility solver returns routes and directions but performs the analysis more slowly than the OD cost matrix solver. If you need driving directions or true shapes of routes, use the closest facility solver; otherwise, use the OD cost matrix solver to reduce the computation time (2).
Methods
The ArcGIS API for Python provides two ways to solve a OD Cost matrix problem, which as shown in the table below:
Operation | network.analysis | features.use_proximity |
---|---|---|
Route | find_routes | plan_routes |
ServiceArea | generate_service_areas | create_drive_time_areas |
ClosestFacility | find_closest_facilities | find_nearest |
OD Cost Matrix | generate_origin_destination_cost_matrix | connect_origins_to_destinations |
These two methods are defined in different modules of the arcgis package, and will make distinct REST calls in the back end. A key separation from network.analysis
to features.use_proximity
is that the former provides full capabilities of solvers and runs faster, and the latter is workflow-driven and provides service-to-service I/O approach.
We will walk through each one of these implementations and further explore the differences in the process. Getting started, let's first connect to a GIS instance.
Connect to GIS object
from arcgis.gis import GIS
import arcgis.network as network
from arcgis.features import FeatureLayer, Feature, FeatureSet, FeatureCollection, use_proximity
import pandas as pd
import time
import datetime as dt
If you have already set up a profile to connect to your ArcGIS Online organization, execute the cell below to load the profile and create the GIS class object. If not, use a traditional username/password log-in e.g. my_gis = GIS('https://www.arcgis.com', 'username', 'password', verify_cert=False, set_active=True)
my_gis = GIS('home')
Method 1 - using arcgis.network.analysis.generate_origin_destination_cost_matrix
Problem statement
Part 5 focuses on how to measure the cost of traveling from facilities to the incidents. Let's assume the first user story is like this:
Jim is an operator working for Medical Emergency Response Office, and needs to dispatch ambulance from nearby hospitals to the spot of incident. Now given the three incidents reported in Redlands and Loma Linda, is Jim able to provide an origin-destination cost matrix?
Now that Jim's objectives are defined, we can go onto break down the problem:
- Data: where to access the input datasets
- Methods: what tools can be used to build the network model and perform OD Cost Matrix analysis
- Tables and maps: deliverables in which directions and routes are visualized.
Let's first access and/or explore the input datasets (in this case, the origins and destinations feature class).
Define Origins and Destinations Feature Class
Generate Origin Destination Cost Matrix
can be associated with a local network dataset or a network service hosted in ArcGIS Online or ArcGIS Enterprise. Here, we will be using an existing feature layer that contains hospitals derived from various sources (refer SOURCE field) for the Homeland Infrastructure Foundation-Level Data (HIFLD) database (https://gii.dhs.gov/HIFLD), which can be accessed from esri_livingatlas
.
If you do not have access to the hospital layer provided by Esri_livingatlas
as referenced in the cell below, an alternative approach is for you to download the hospital listing of San Bernadino County from this source and publish the csv to the organization before proceeding forward.
""" This try-except block will help you download the CSV and publish to current GIS object, if
"You do not have permissions to access this resource or perform this operation."
"""
try:
hospital_item = my_gis.content.get("a2817bf9632a43f5ad1c6b0c153b0fab")
except RuntimeError as ne:
try:
print("Trying from an alternative source...")
hospital_item = my_gis.content.get("50fb63f303304835a048d16bd86c3024")
except RuntimeError as ne:
print("Trying to publish from csv...")
import requests
import csv
import os
out_file_name = 'hospitals_SB_county.csv'
url = "https://data.chhs.ca.gov/datastore/dump/641c5557-7d65-4379-8fea-6b7dedbda40b?q=&sort=_id+asc&fields=OSHPD_ID%2CFACILITY_NAME%2CLICENSE_NUM%2CFACILITY_LEVEL_DESC%2CDBA_ADDRESS1%2CDBA_CITY%2CDBA_ZIP_CODE%2CCOUNTY_CODE%2CCOUNTY_NAME%2CER_SERVICE_LEVEL_DESC%2CTOTAL_NUMBER_BEDS%2CFACILITY_STATUS_DESC%2CFACILITY_STATUS_DATE%2CLICENSE_TYPE_DESC%2CLICENSE_CATEGORY_DESC%2CLATITUDE%2CLONGITUDE&filters=%7B%22COUNTY_CODE%22%3A+%5B%2236%22%5D%7D&format=csv"
download = requests.get(url)
with open(out_file_name, 'w') as out_file:
out_file.writelines(download.text)
print(out_file_name)
csv_item = my_gis.content.add({}, out_file_name)
hospital_item = csv_item.publish()
display(hospital_item)
You do not have permissions to access this resource or perform this operation.
Trying from an alternative source...
hospital_fl = FeatureLayer(hospital_item.url + "/0")
""" If you are using the exisiting layer from Esri_LivngAtlas, there is a "County" column in the dataset;
or else, the feature layer collection published from the downloaded CSV file is already targetted at SB County.
"""
try:
facilities = hospital_fl.query(where="County='SAN BERNARDINO' AND State='CA'", as_df=False)
except RuntimeError as re:
""" when seeing 'Invalid field: County' parameter is invalid
"""
print("Trying from an alternative approach...")
facilities = hospital_fl.query(where="Dba_city='REDLANDS' or Dba_city='LOMA LINDA'", as_df=False)
display(facilities)
'Invalid field: County' parameter is invalid
Trying from an alternative approach...
<FeatureSet> 33 features
Now we have the facilities layer
ready, we can go onto define the incidents layer
. Here, we randomly picked six locations in Redlands, CA.
incidents_json = {
"features": [{"attributes": {"CurbApproach": 0,
"ID": "C100045",
"Name": "Incident at Esri"},
"geometry": {"x": -117.19569523299998, "y": 34.05608640000003}},
{"attributes": {"CurbApproach": 0,
"ID": "F100086",
"Name": "Incident at APT"},
"geometry": {"x": -117.20520037855628, "y": 34.04472649163186}},
{"attributes": {"CurbApproach": 0,
"ID": "C100097",
"Name": "Incident at Walmart"},
"geometry": {"x": -117.222253, "y": 34.065378}},
{"attributes": {"CurbApproach": 0,
"ID": "C100097",
"Name": "Incident at High school"},
"geometry": {"x": -117.192296, "y": 34.060759}},
{"attributes": {"CurbApproach": 0,
"ID": "C100097",
"Name": "Incident at Bowling"},
"geometry": {"x": -117.194699, "y": 34.063568}},
{"attributes": {"CurbApproach": 0,
"ID": "C100097",
"Name": "Incident at Burger"},
"geometry": {"x": -117.201201, "y": 34.063364}}],
"spatialReference": {"wkid": 4326, "latestWkid": 4326},
"geometryType": "esriGeometryPoint",
"fields" : [
{"name" : "ID", "type" : "esriFieldTypeString", "alias" : "ID", "length" : "50"},
{"name" : "Name", "type" : "esriFieldTypeString", "alias" : "Name", "length" : "50"},
{"name" : "CurbApproach", "type" : "esriFieldTypeInteger", "alias" : "CurbApproach"}
]
}
incidents = FeatureSet.from_dict(incidents_json)
Let's have the two layers visualized in the map view.
map1 = my_gis.map('Redlands, CA', zoomlevel=12)
map1

map1.clear_graphics()
hospital_symbol = {"type":"esriPMS",
"url":"http://static.arcgis.com/images/Symbols/SafetyHealth/Hospital.png",
"contentType": "image/png", "width":20, "height":20}
map1.draw(facilities, symbol=hospital_symbol)
traffic_accident_symbol = {"type":"esriPMS",
"url":"http://static.arcgis.com/images/Symbols/Transportation/TrafficAccident.png",
"contentType": "image/png", "width":20, "height":20}
map1.draw(incidents, symbol=traffic_accident_symbol)
Solving Problem
What's worth mentioning is that, in using generate_origin_destination_cost_matrix
, 0.0005 credits per input origin and destination pair will be charged. For example, if there are 100 origins and 200 destinations, the cost will be 10 credits. If you specify a cutoff or limit the number of destinations, for instance, to find only 5 closest destinations within 10 minutes of every origin, the cost will still be 10 credits, as the credits depend on the number of input origin destination pairs.
-
TargetDestinationCount
- The maximum number of destinations that must be found for the origin. If a value is not specified, the value from the Number of Destinations to Find parameter is used. -
Cutoff
- Specify the travel time or travel distance value at which to stop searching for destinations from the origin. Any destination beyond the cutoff value will not be considered. The value needs to be in the units specified by the Time Units parameter if the impedance attribute in your travel mode is time based or in the units specified by the Distance Units parameter if the impedance attribute in your travel mode is distance based. If a value is not specified, the tool will not enforce any travel time or travel distance limit when searching for destinations. -
Specify
origin_destination_line_shape
to see the output in map. The resulting lines of an OD cost matrix can be represented with either straight-line geometry or no geometry at all. Even though the lines are straight for performance reasons, they always store the travel time and travel distance along the street network, not straight-line distance.
Note: You can set save_output_network_analysis_layer
to True if you want to output the resulting NA layer as Layer file, though this process can take up more computation time (4).
%%time
current_time= dt.datetime.now()
result1 = network.analysis.generate_origin_destination_cost_matrix(origins=incidents, destinations=facilities,
cutoff=10, time_of_day=current_time,
number_of_destinations_to_find=4,
origin_destination_line_shape='Straight Line',
gis=my_gis)
Wall time: 8.35 s
Now, check if the tool is run successfully, and what are types of the elements in the returned result set, and also get the output network analysis layer's url.
print('Analysis succeeded? {}'.format(result1.solve_succeeded))
Analysis succeeded? True
display(result1.output_origin_destination_lines,result1.output_origins, result1.output_destinations)
<FeatureSet> 24 features
<FeatureSet> 6 features
<FeatureSet> 33 features
Tabularizing the response from generate_origin_destination_cost_matrix
Now, let's see the output lines table.
result1.output_origin_destination_lines.sdf.tail()
DestinationName | DestinationOID | DestinationRank | ObjectID | OriginName | OriginOID | SHAPE | Shape_Length | Total_Distance | Total_Time | |
---|---|---|---|---|---|---|---|---|---|---|
19 | Location 4 | 23 | 4 | 20 | Incident at Bowling | 5 | {'paths': [[[-117.19469899999996, 34.063568000... | 0.014748 | 1.909752 | 4.016058 |
20 | Location 31 | 357 | 1 | 21 | Incident at Burger | 6 | {'paths': [[[-117.20120099999997, 34.063364000... | 0.001528 | 0.202159 | 0.665024 |
21 | Location 27 | 245 | 2 | 22 | Incident at Burger | 6 | {'paths': [[[-117.20120099999997, 34.063364000... | 0.005566 | 0.684698 | 1.734902 |
22 | Location 28 | 256 | 3 | 23 | Incident at Burger | 6 | {'paths': [[[-117.20120099999997, 34.063364000... | 0.005566 | 0.684698 | 1.734902 |
23 | Location 5 | 41 | 4 | 24 | Incident at Burger | 6 | {'paths': [[[-117.20120099999997, 34.063364000... | 0.016599 | 2.150643 | 3.899969 |
# filter only the required columns
od_df = result1.output_origin_destination_lines.sdf[['OriginName', 'OriginOID','DestinationOID','Total_Distance','Total_Time']]
display(od_df)
OriginName | OriginOID | DestinationOID | Total_Distance | Total_Time | |
---|---|---|---|---|---|
0 | Incident at Esri | 1 | 245 | 1.103517 | 2.071802 |
1 | Incident at Esri | 1 | 256 | 1.103517 | 2.071802 |
2 | Incident at Esri | 1 | 357 | 1.229031 | 2.671727 |
3 | Incident at Esri | 1 | 23 | 1.710835 | 3.592510 |
4 | Incident at APT | 2 | 65 | 0.692399 | 1.274009 |
5 | Incident at APT | 2 | 41 | 0.431708 | 1.310646 |
6 | Incident at APT | 2 | 90 | 1.093573 | 1.947980 |
7 | Incident at APT | 2 | 98 | 1.332103 | 2.174232 |
8 | Incident at Walmart | 3 | 121 | 1.269695 | 3.007999 |
9 | Incident at Walmart | 3 | 207 | 1.413950 | 3.194782 |
10 | Incident at Walmart | 3 | 357 | 2.099291 | 4.401875 |
11 | Incident at Walmart | 3 | 48 | 1.978585 | 4.644457 |
12 | Incident at High school | 4 | 245 | 1.295173 | 2.218085 |
13 | Incident at High school | 4 | 256 | 1.295173 | 2.218085 |
14 | Incident at High school | 4 | 357 | 1.285388 | 2.442592 |
15 | Incident at High school | 4 | 23 | 1.269365 | 2.524285 |
16 | Incident at Bowling | 5 | 357 | 0.819273 | 2.058579 |
17 | Incident at Bowling | 5 | 245 | 1.137488 | 2.551424 |
18 | Incident at Bowling | 5 | 256 | 1.137488 | 2.551424 |
19 | Incident at Bowling | 5 | 23 | 1.909752 | 4.016058 |
20 | Incident at Burger | 6 | 357 | 0.202159 | 0.665024 |
21 | Incident at Burger | 6 | 245 | 0.684698 | 1.734902 |
22 | Incident at Burger | 6 | 256 | 0.684698 | 1.734902 |
23 | Incident at Burger | 6 | 41 | 2.150643 | 3.899969 |
We need to change the format to get a matrix with rows as origins and columns as destinations, with impedance value as travel time or travel distance. We will use the pivot_table
feature of Pandas to accomplish that.
Note that number_of_destinations_to_find
is set to 4, so for the destinations that are not within the top 4 closest to the origins, the total_distance
will shows as NaN
in the output table below:
# user pivot_table
od_pivot = od_df.pivot_table(index='OriginOID', columns='DestinationOID')
od_pivot
Total_Distance | ... | Total_Time | |||||||||||||||||||
---|---|---|---|---|---|---|---|---|---|---|---|---|---|---|---|---|---|---|---|---|---|
DestinationOID | 23 | 41 | 48 | 65 | 90 | 98 | 121 | 207 | 245 | 256 | ... | 41 | 48 | 65 | 90 | 98 | 121 | 207 | 245 | 256 | 357 |
OriginOID | |||||||||||||||||||||
1 | 1.710835 | NaN | NaN | NaN | NaN | NaN | NaN | NaN | 1.103517 | 1.103517 | ... | NaN | NaN | NaN | NaN | NaN | NaN | NaN | 2.071802 | 2.071802 | 2.671727 |
2 | NaN | 0.431708 | NaN | 0.692399 | 1.093573 | 1.332103 | NaN | NaN | NaN | NaN | ... | 1.310646 | NaN | 1.274009 | 1.94798 | 2.174232 | NaN | NaN | NaN | NaN | NaN |
3 | NaN | NaN | 1.978585 | NaN | NaN | NaN | 1.269695 | 1.41395 | NaN | NaN | ... | NaN | 4.644457 | NaN | NaN | NaN | 3.007999 | 3.194782 | NaN | NaN | 4.401875 |
4 | 1.269365 | NaN | NaN | NaN | NaN | NaN | NaN | NaN | 1.295173 | 1.295173 | ... | NaN | NaN | NaN | NaN | NaN | NaN | NaN | 2.218085 | 2.218085 | 2.442592 |
5 | 1.909752 | NaN | NaN | NaN | NaN | NaN | NaN | NaN | 1.137488 | 1.137488 | ... | NaN | NaN | NaN | NaN | NaN | NaN | NaN | 2.551424 | 2.551424 | 2.058579 |
6 | NaN | 2.150643 | NaN | NaN | NaN | NaN | NaN | NaN | 0.684698 | 0.684698 | ... | 3.899969 | NaN | NaN | NaN | NaN | NaN | NaN | 1.734902 | 1.734902 | 0.665024 |
6 rows × 22 columns
If you want to export the pivot table into a file, use to_csv
to save as file-based csv output.
od_pivot.to_csv('data/OD_Matrix.csv')
Visualizing the response from generate_origin_destination_cost_matrix
With the customized symbologies defined here, output_origin_destination_lines
can also be rendered on the map view:
""" Define a list of colors/styles for routes;
alternatively, use "'type': 'simple-line', 'style': 'solid'"
"""
allocation_line_symbol = [{'type': 'esriSLS', 'style': 'esriSLSSolid',
'color': [237, 17, 189, 100], 'width': 6},
{'type': 'esriSLS', 'style': 'esriSLSSolid',
'color': [30, 139, 235, 90], 'width': 6},
{'type': 'esriSLS', 'style': 'esriSLSSolid',
'color': [66, 240, 43, 80], 'width': 6},
{'type': 'esriSLS', 'style': 'esriSLSSolid',
'color': [240, 234, 67, 70], 'width': 6}]
names_list = ["Incident at Esri",
"Incident at APT",
"Incident at Walmart",
"Incident at High school",
"Incident at Bowling",
"Incident at Burger"]
map2 = my_gis.map('Redlands, CA', zoomlevel=13)
map2.basemap = "dark-gray"
map2
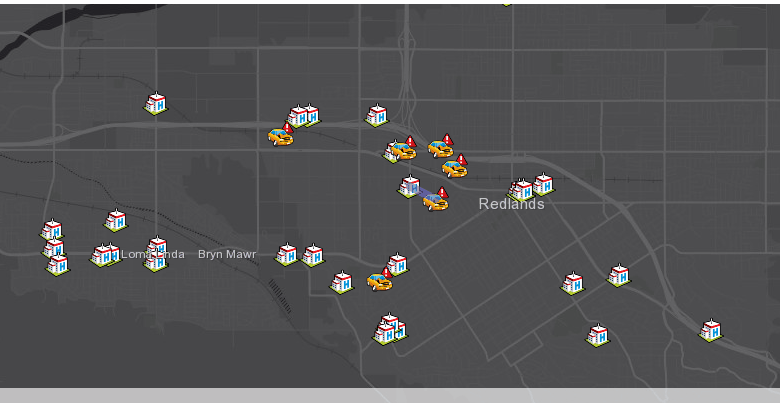
map2.draw(result1.output_destinations, symbol=hospital_symbol)
map2.draw(result1.output_origins, symbol=traffic_accident_symbol)
count1 = 0
count2 = 0
for route in result1.output_origin_destination_lines:
route_name = route.get_value("OriginName") + " ---> " + route.get_value("DestinationName")
print(route_name)
#get the name
name = route.attributes['OriginName']
#get the color
fill_symbol=allocation_line_symbol[count1%4]
if names_list[0] in name:
count1+=1
else:
fill_symbol=allocation_line_symbol[count2%4]
count2+=1
#set popup
popup={"title": "OD cost matrix {}".format(route_name),
"content": "{} minutes".format(route.attributes['Total_Time'])}
map2.draw(route.geometry, symbol=fill_symbol, popup=popup, attributes={"title": route_name})
time.sleep(2)
Incident at Esri ---> Location 27 Incident at Esri ---> Location 28 Incident at Esri ---> Location 31 Incident at Esri ---> Location 4 Incident at APT ---> Location 5 Incident at APT ---> Location 12 Incident at APT ---> Location 16 Incident at APT ---> Location 30 Incident at Walmart ---> Location 23 Incident at Walmart ---> Location 26 Incident at Walmart ---> Location 6 Incident at Walmart ---> Location 31 Incident at High school ---> Location 27 Incident at High school ---> Location 28 Incident at High school ---> Location 31 Incident at High school ---> Location 4 Incident at Bowling ---> Location 31 Incident at Bowling ---> Location 27 Incident at Bowling ---> Location 28 Incident at Bowling ---> Location 4 Incident at Burger ---> Location 31 Incident at Burger ---> Location 27 Incident at Burger ---> Location 28 Incident at Burger ---> Location 5
The map view can be also saved into a web map for future references.
item_properties = {
"title": "OD Cost Matrix from incidents to hospitals in San Bernadino County",
"tags" : "OD Cost Matrix",
"snippet": "OD Cost Matrix from incidents to hospitals in San Bernadino County",
"description": "a web map of OD Cost Matrix from incidents to hospitals in San Bernadino County"
}
item = map2.save(item_properties)
item
We have been using the generate_origin_destination_cost_matrix
tool in the network.analysis
module up to this point. From now on, let's use a different method - connect_origins_to_destinations
- defined in the features.use_proximity
module, to achieve a workflow driven, Feature Service to Feature Service user experience.
Method 2 - using arcgis.features.use_proximity.connect_origins_to_destinations
Quick facts about the tool
The Connect Origins to Destinations
task measures the travel time or distance between pairs of points. Using this tool, you can
- Calculate the total distance or time commuters travel on their home-to-work trips.
- Measure how far customers are traveling to shop at your stores. Use this information to define your market reach, especially when targeting advertising campaigns or choosing new store locations.
- Calculate the expected trip mileage for your fleet of vehicles. Afterward, run the Summarize Within tool to report mileage by state or other region.
You provide starting and ending points, and the tool returns a layer containing route lines, including measurements, between the paired origins and destinations.
Problem Statement
Now say Jim has changed his job and become a logistics analyst for a shipping company, and he needs to look into the OD cost matrix from where his company is at (Detroit, MI) to seven other cities in the Lake Area. What would Jim do to perform his day-to-day scheduling?
First, Jim needs to set down the origins and destinations layers for the transportation demands.
Define Origins and Destinations Layers
Get access to the existing feature service titled "USA Major Cities":
sample_cities = my_gis.content.search('title:"USA Major Cities" type:Feature Service',
outside_org=True)[0]
sample_cities
cities_fl = FeatureLayer(sample_cities.url + "/0")
type(cities_fl)
arcgis.features.layer.FeatureLayer
The cities that all the goodies will be transported to are:
stops_cities = ['Chicago', 'Indiannapolis', 'Cleveland',
'Columbus', 'pittsburgh', 'Buffalo']
values = "'" + "', '".join(stops_cities) + "'"
stops_layer = {'url': sample_cities.layers[0].url,
'filter': "ST in ('IL', 'IN', 'OH', 'PA', 'NY') AND NAME IN ({0})".format(values)}
end_cities_fset = cities_fl.query(where="ST in ('WI', 'IL', 'IN', 'OH', 'PA', 'NY') AND NAME IN ({0})".format(values), as_df=False)
end_cities_fset
<FeatureSet> 6 features
Where the transportation get started is:
start_layer = {'url': sample_cities.layers[0].url,
'filter': "ST in ('MI') AND NAME IN ('Detroit')"}
start_cities_fset = cities_fl.query(where="ST in ('MI') AND NAME IN ('Detroit')", as_df=False)
start_cities_fset
<FeatureSet> 1 features
Let's first look at the map with starting point (Detroit, being signified as red balloon point A), and the other six ending points (as signified as in green balloon point B):
map4a = my_gis.map('Detriot, MI', zoomlevel=8)
map4a

map4a.clear_graphics()
start_symbol = {"angle":0,"xoffset":0,"yoffset":8.15625,"type":"esriPMS",
"url":"http://static.arcgis.com/images/Symbols/AtoZ/redA.png",
"contentType":"image/png","width":15.75,"height":21.75}
end_symbol = {"angle":0,"xoffset":0,"yoffset":8.15625,"type":"esriPMS",
"url":"http://static.arcgis.com/images/Symbols/AtoZ/greenB.png",
"contentType":"image/png","width":15.75,"height":21.75}
map4a.draw(start_cities_fset, symbol=start_symbol)
map4a.draw(end_cities_fset, symbol=end_symbol)
Next, we will use the solver to calculate the OD cost matrix, and visualize the routing paths from A to B.
Solving Problem (With output_name specified)
With output_name
specified, the return type is a Feature Layer Collection
Item, and the layer containing route lines, including measurements, between the paired origins and destinations.
Other important parameters include:
origins_layer
: Required layer. The starting point or points of the routes to be generated.destinations_layer
: Required layer. The routes end at points in the destinations layer.measurement_type
: String. The origins and destinations can be connected by measuring straight-line distance, or by measuring travel time or travel distance along a street network using various modes of transportation known as travel modes. Valid values are a string, "StraightLine", which indicates Euclidean distance to be used as distance measure or a Python dictionary representing settings for a travel mode chosen from [‘Driving Distance’, ‘Driving Time’, ‘Rural Driving Distance’, ‘Rural Driving Time’, ‘Trucking Distance’, ‘Trucking Time’, ‘Walking Distance’, ‘Walking Time’]. The default option isDrivingTime
.
Worth mentioning here is that, when measurement_type
set to "StraightLine", not only the representation in the output analysis layer is a straight line, but also the calculation of distance is based off the Euclidean distance of the straight line. Unlike generate_origin_destination_cost_matrix
in network.analysis
, the resulting connection line is represented as generalized straight lines but the real cost paths being calculated and stored are along the network routes.
%%time
result2 = use_proximity.connect_origins_to_destinations(origins_layer=start_layer,
destinations_layer=stops_layer,
context={'outSR': {"wkid": 4326}},
time_of_day=current_time,
output_name="OD Cost Matrix (Oct 2019)",
gis=my_gis)
Network elements with avoid-restrictions are traversed in the output (restriction attribute names: "Avoid Unpaved Roads" "Through Traffic Prohibited").
Wall time: 51.4 s
result2
The first sublayer of result2
is the nearest facilities, and the second sublayer is the route.
od_cost_matrix_sublayer = FeatureLayer.fromitem(result2, layer_id=0)
od_cost_matrix_sublayer.url
'https://services7.arcgis.com/JEwYeAy2cc8qOe3o/arcgis/rest/services/OD Cost Matrix (Oct 2019)/FeatureServer/0'
od_df = od_cost_matrix_sublayer.query(where='1=1', as_df=True)
# filter only the required columns
od_df2 = od_df[['RouteName','OriginOID','DestinationOID','Total_Miles','StartTime','EndTime','Total_Minutes']]
od_df2.tail(6)
RouteName | OriginOID | DestinationOID | Total_Miles | StartTime | EndTime | Total_Minutes | |
---|---|---|---|---|---|---|---|
0 | Route 1 - Detroit - Chicago | 1533 | 63 | 275.444212 | 2019-10-24 19:56:17 | 2019-10-24 23:17:48 | 261.514763 |
1 | Route 2 - Detroit - Columbus | 1533 | 256 | 326.222952 | 2019-10-24 19:56:17 | 2019-10-25 01:06:55 | 310.638586 |
2 | Route 3 - Detroit - Buffalo | 1533 | 2075 | 259.663696 | 2019-10-24 19:56:17 | 2019-10-25 00:28:21 | 272.074868 |
3 | Route 4 - Detroit - Cleveland | 1533 | 2385 | 168.146931 | 2019-10-24 19:56:17 | 2019-10-24 22:41:58 | 165.690078 |
4 | Route 5 - Detroit - Columbus | 1533 | 256 | 199.968721 | 2019-10-24 19:56:17 | 2019-10-24 23:18:16 | 201.984500 |
5 | Route 6 - Detroit - Pittsburgh | 1533 | 2989 | 285.264445 | 2019-10-24 19:56:17 | 2019-10-25 00:27:18 | 271.010365 |
As shown here, the six routes from origin (Detroit) to destinations (Chicago, Columbus, etc.) are shown in the matrix above, with start_time, end_time, total_minutes and total_miles. We can also pivot the table, and summarize these six routes with their sole "total_minutes" attribute.
# user pivot_table
od_pivot = od_df2.pivot_table(index='OriginOID', columns='DestinationOID')
od_pivot
Total_Miles | Total_Minutes | |||||||||
---|---|---|---|---|---|---|---|---|---|---|
DestinationOID | 63 | 256 | 2075 | 2385 | 2989 | 63 | 256 | 2075 | 2385 | 2989 |
OriginOID | ||||||||||
1533 | 275.444212 | 263.095837 | 259.663696 | 168.146931 | 285.264445 | 261.514763 | 256.311543 | 272.074868 | 165.690078 | 271.010365 |
As in map4a
rendered above with the origin and the destinations, we will now go on to add the routes to the map view. Note that the origin and destinations are symbolized as FeatureSet, while the resulting routes are added to the map view as Feature Layer.
map4a.add_layer(result2)
Now we have seen how a Feature Service (input) to Feature service (output) works, let's explore a more traditional way of solving/parsing/tabularizing/drawing user experience.
Solving Problem (Without output_name specified)
Without specifying output_name
in the argument list, the return type is a dictionary of four entries:
%%time
result2b = use_proximity.connect_origins_to_destinations(origins_layer=start_layer,
destinations_layer=stops_layer,
context={'outSR': {"wkid": 4326}},
time_of_day=current_time,
gis=my_gis)
Network elements with avoid-restrictions are traversed in the output (restriction attribute names: "Avoid Unpaved Roads" "Through Traffic Prohibited").
Wall time: 37.9 s
result2b
{'routes_layer': <FeatureCollection>, 'unassigned_origins_layer': '', 'unassigned_destinations_layer': '', 'route_layer_items': ''}
Looking at the time lapse taken in the execution of the previous cell, solver without outputting a feature layer works much faster (37.9 seconds compared to 51.4 seconds).
Tabularizing the response from connect_origins_to_destinations
We then take advantage of the routes_layer
component of the returned object in creating a pandas dataframe displaying the OD cost matrix for Detroit to six destinations.
df5 = result2b["routes_layer"].query().sdf
df5[['RouteName','OriginOID','DestinationOID','Total_Miles','StartTime','EndTime','Total_Minutes']]
RouteName | OriginOID | DestinationOID | Total_Miles | StartTime | EndTime | Total_Minutes | |
---|---|---|---|---|---|---|---|
0 | Route 1 - Detroit - Chicago | 1533 | 63 | 275.444212 | 2019-10-31 23:11:30 | 2019-11-01 02:24:14.476999998 | 252.741290 |
1 | Route 2 - Detroit - Columbus | 1533 | 256 | 322.114356 | 2019-10-31 23:11:30 | 2019-11-01 04:15:55.417999983 | 304.423627 |
2 | Route 3 - Detroit - Buffalo | 1533 | 2075 | 259.663696 | 2019-10-31 23:11:30 | 2019-11-01 03:38:20.026000023 | 266.833772 |
3 | Route 4 - Detroit - Cleveland | 1533 | 2385 | 168.146931 | 2019-10-31 23:11:30 | 2019-11-01 01:49:56.456000090 | 158.440937 |
4 | Route 5 - Detroit - Columbus | 1533 | 256 | 199.968721 | 2019-10-31 23:11:30 | 2019-11-01 02:25:21.056999922 | 193.850944 |
5 | Route 6 - Detroit - Pittsburgh | 1533 | 2989 | 285.264445 | 2019-10-31 23:11:30 | 2019-11-01 03:36:11.479000092 | 264.691321 |
Visualizing the response from connect_origins_to_destinations
First create the map view, then customize the symbologies for the service areas and the facilities, and finally render the routes and stops.
map4b = my_gis.map('Detroit, MI', zoomlevel=8)
map4b.basemap = 'dark-gray'
map4b

map4b.draw(start_cities_fset, symbol=start_symbol)
map4b.draw(end_cities_fset, symbol=end_symbol)
count1 = 0
count2 = 0
for route in result2b["routes_layer"].query():
#get the name
name = route.attributes['RouteName']
#get the color
fill_symbol=allocation_line_symbol[count1%4]
if names_list[0] in name:
count1+=1
else:
fill_symbol=allocation_line_symbol[count2%4]
count2+=1
#set popup
popup={"title": "OD cost matrix {}".format(route.attributes['RouteName']),
"content": "{} minutes".format(route.attributes['Total_Minutes'])}
map4b.draw(route.geometry, symbol=fill_symbol, popup=popup, attributes={"title":route.attributes['RouteName']})
time.sleep(2)
item_properties["title"] = "OD Cost matrix from Detriot to nearby major cities"
item = map4b.save(item_properties)
item
In the last section of this guide, we have adopted a different method - arcgis.features.use_proximity.connect_origins_to_destinations
- in creating OD cost matrix. In doing so, we also explored the two scenarios with output_name specified (which forms a Feature Service to Feature Service user experience), and a more traditional compute/parse/draw approach when output_name is not present.
Conclusions
This part of network Analysis Guide demonstrated how you can constuct an OD cost matrix using the ArcGIS API for Python. We started by defining origins and destinations layers, then used the generate_origin_destination_cost_matrix()
method under the arcgis.network.analysis
module, and/or the use_proximity.connect_origins_to_destinations()
method under arcgis.features
to compute the OD cost matrix.
The OD cost matrix becomes an important analytical output for downstream routing and other network analysis problems. Imagine you run a pizza restaurant and receive orders for delivery in a central location. Based on the distance to these demand points, you need to decide which supply point (pizza place) should service which demand point (customer address). You can solve problems such as these by computing the OD cost matrix.
References
[1] "Tutorial: Generate Origin Destination Cost Matrix", https://pro.arcgis.com/en/pro-app/help/analysis/networks/od-cost-matrix-tutorial.htm, accessed 10/07/2019
[2] "OD cost matrix analysis layer", https://pro.arcgis.com/en/pro-app/help/analysis/networks/od-cost-matrix-analysis-layer.htm, accessed on 10/07/2019
[3] "OD cost matrix analysis", https://desktop.arcgis.com/en/arcmap/latest/extensions/network-analyst/od-cost-matrix.htm, accessed on 10/07/2019
[4] "Calculating Origin Destinations nXn Matrix given set of origins and destinations", https://developers.arcgis.com/python/sample-notebooks/calculating-nxn-od-cost-matrix/, accessed on 10/09/2019