What is clustering?
Clustering is a method of reducing points in a layer by grouping them into clusters based on their spatial proximity to one another. Typically, clusters are proportionally sized based on the number of features within each cluster.
This is an effective way to show areas where many points stack on top of one another.
Why is clustering useful?
Large point layers can be deceptive. What appears to be just a few points can in reality be several thousand. Clustering allows you to visually represent large numbers of points in relatively small areas.
For example, the following map shows the locations of thousands of power plants. In the image below, regions A and B both have a high density of points, making them impossible to compare.
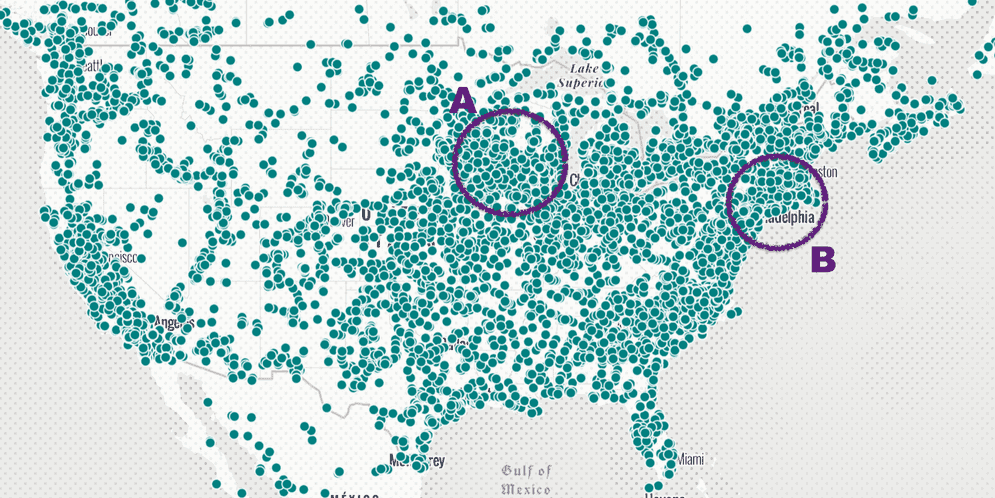
However, when clustering is enabled, the user can now clearly see that region B has nearly twice as many points as region A.
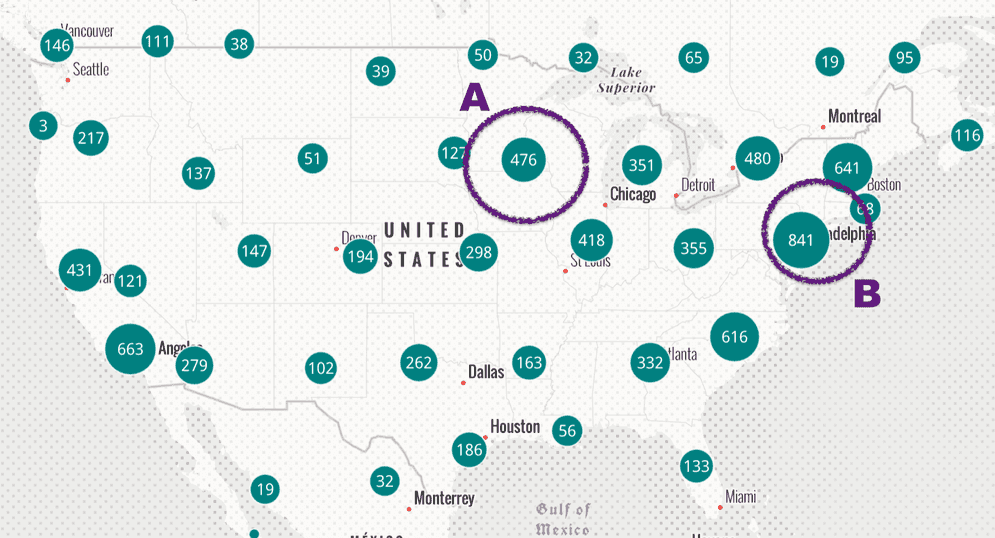
How clustering works
Clustering is configured on the featureReduction property of the layer. You can enable clustering with minimal code by setting the feature
type to cluster
.
layer.featureReduction = {
type: "cluster"
};
The feature
property gives you control over many other cluster properties. The clusterRadius defines each cluster's area of influence for including features. You may also define popupTemplates and labels for clusters to summarize the features included in the cluster.
Examples
Basic clustering
The following example demonstrates how to enable clustering on a point layer and configure labels and a popup for displaying the cluster count.
The aggregate fields used by clusters are generated once clustering is enabled on the layer. By default, all clustered layers have a cluster_
aggregate field. This can be used in the labels and the popup for each cluster. Other fields used in the layer's renderer may be accessible for display in the popup. You can learn more about how to use these in the FeatureReductionCluster.popupTemplate documentation.
See the Related samples and resources below for more examples of how to summarize data within a cluster's popup.
clusteredLayer.featureReduction = {
type: "cluster",
clusterMinSize: 16.5,
// defines the label within each cluster
labelingInfo: [
{
deconflictionStrategy: "none",
labelExpressionInfo: {
expression: "Text($feature.cluster_count, '#,###')"
},
symbol: {
type: "text",
color: "white",
font: {
family: "Noto Sans",
size: "12px"
}
},
labelPlacement: "center-center"
}
],
// information to display when the user clicks a cluster
popupTemplate: {
title: "Cluster Summary",
content: "This cluster represents <b>{cluster_count}</b> features.",
fieldInfos: [{
fieldName: "cluster_count",
format: {
places: 0,
digitSeparator: true
}
}]
}
};
Suggested cluster defaults
By default, the cluster symbol always summarizes the features in the cluster. When a layer has a UniqueValueRenderer, the symbol of each cluster represents the predominant value of features in the cluster. When a layer has any visual variables applied to it, the average of each variable in the cluster is applied to the cluster symbol. The fields describing the predominant type and average of numeric fields can be referenced in the cluster popup and label.
This example uses smart mapping methods to demonstrate how to generate the suggested cluster configuration specific to the layer's renderer.
// generates default popupTemplate
const popupTemplate = await clusterPopupCreator
.getTemplates({ layer })
.then( (popupTemplateResponse) => popupTemplateResponse.primaryTemplate.value );
// generates default labelingInfo
const { labelingInfo, clusterMinSize } = await clusterLabelCreator
.getLabelSchemes({
layer,
view
})
.then((labelSchemes) => labelSchemes.primaryScheme);
// Set this object on layer.featureReduction
return {
type: "cluster",
popupTemplate,
labelingInfo,
clusterMinSize
};
Clusters as pie charts
By default, when a layer has a UniqueValueRenderer or ClassBreaksRenderer, the symbol of each cluster represents the predominant category within the cluster.
You may prefer to visualize clusters of type-based renderers as pie charts rather than the predominant category. To do this, you can call the pieChart.createRendererForClustering method, which will create a pie chart renderer based on the categories defined in a UniqueValueRenderer or a ClassBreaksRenderer.
The fields and renderer returned from this method should be set directly on the FeatureReductionCluster instance on the layer.
const { renderer, fields } = await pieChartRendererCreator.createRendererForClustering({
layer,
shape: "donut"
});
layer.featureReduction = {
type: "cluster",
fields,
renderer
}
Related samples and resources
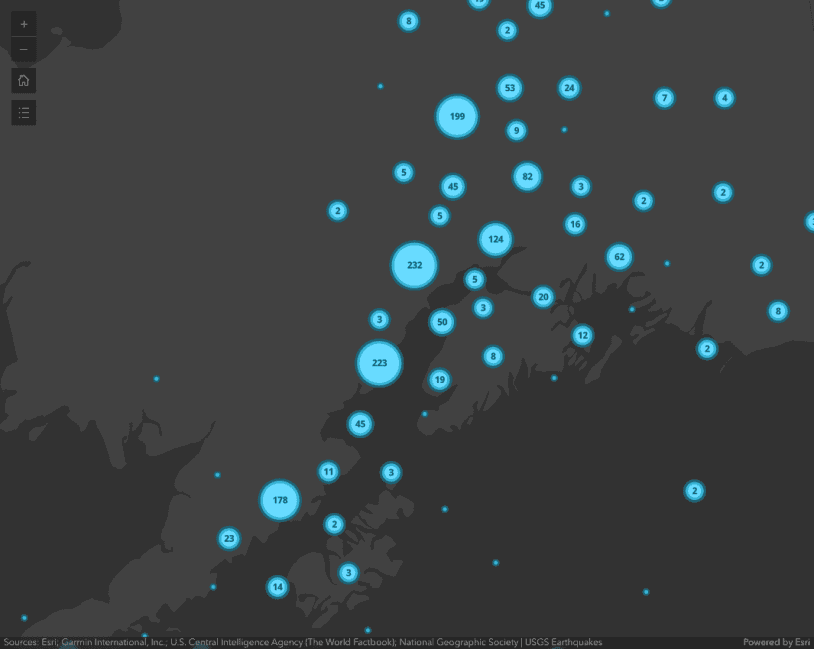
Intro to clustering
Intro to clustering
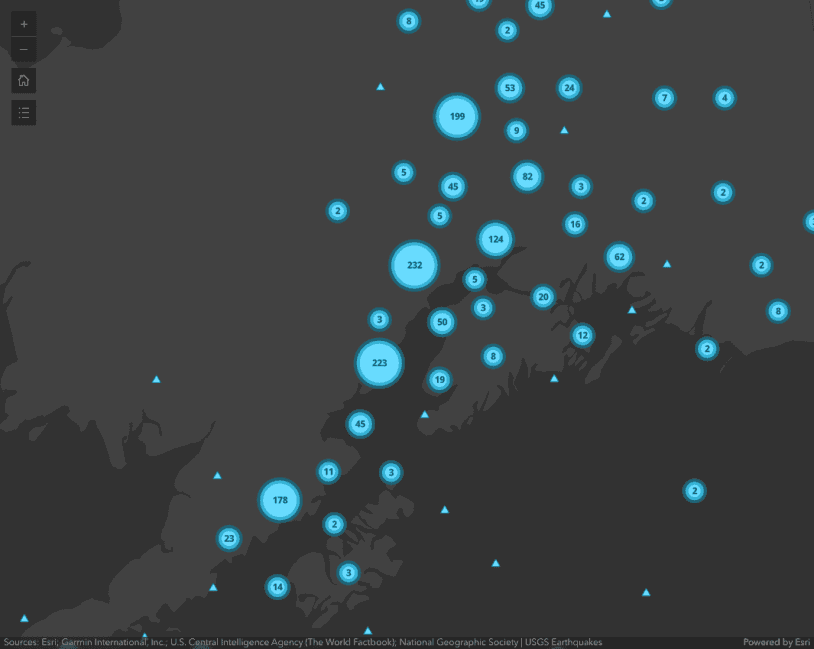
Override cluster symbol
Override cluster symbol
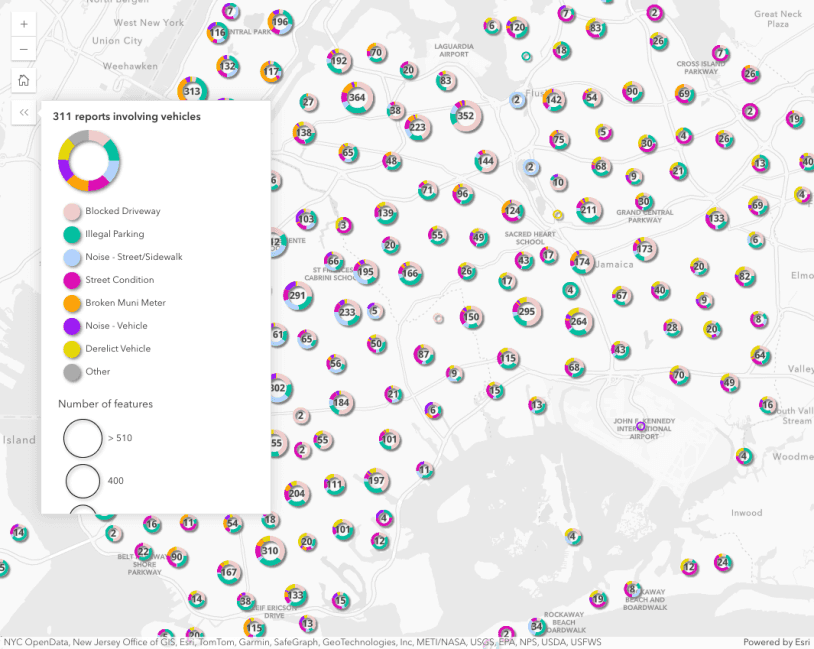
Clusters as pie charts
Clusters as pie charts
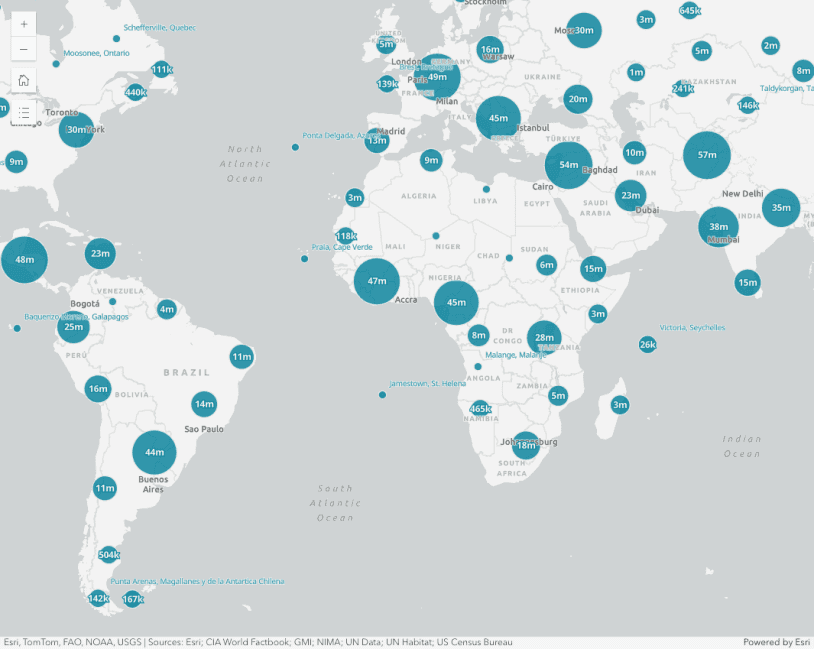
Cluster size based on the sum of a field
Cluster size based on the sum of a field
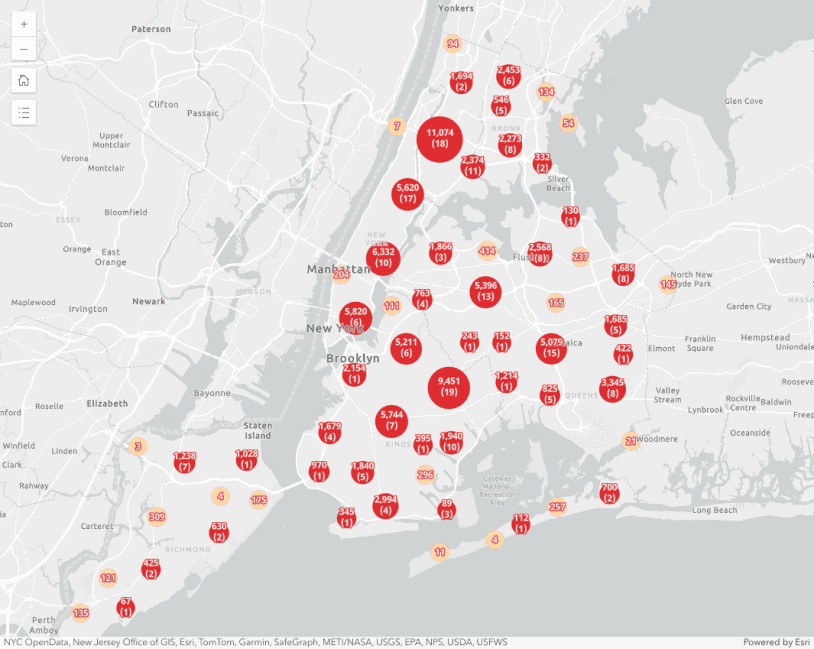
Clustering with aggregate fields
Clustering with aggregate fields
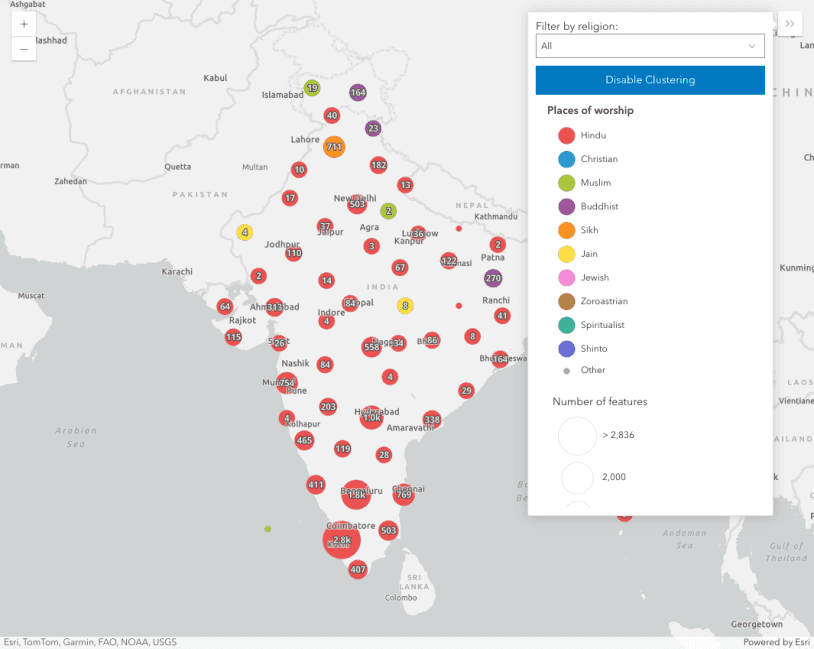
Clustering - generate suggested configuration
Clustering - generate suggested configuration
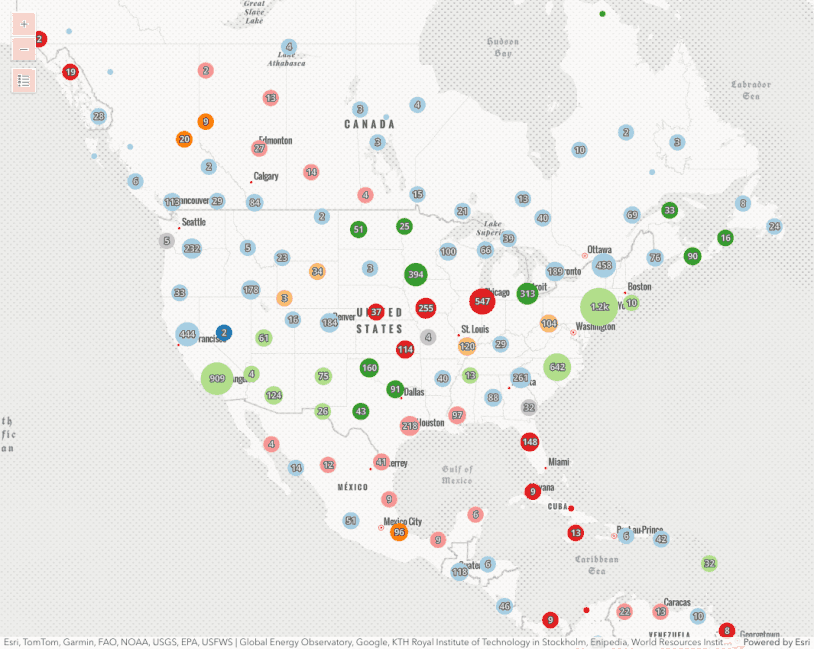
Clustering - filter popup features
This sample demonstrates how to filter clustered features within a cluster's popup.
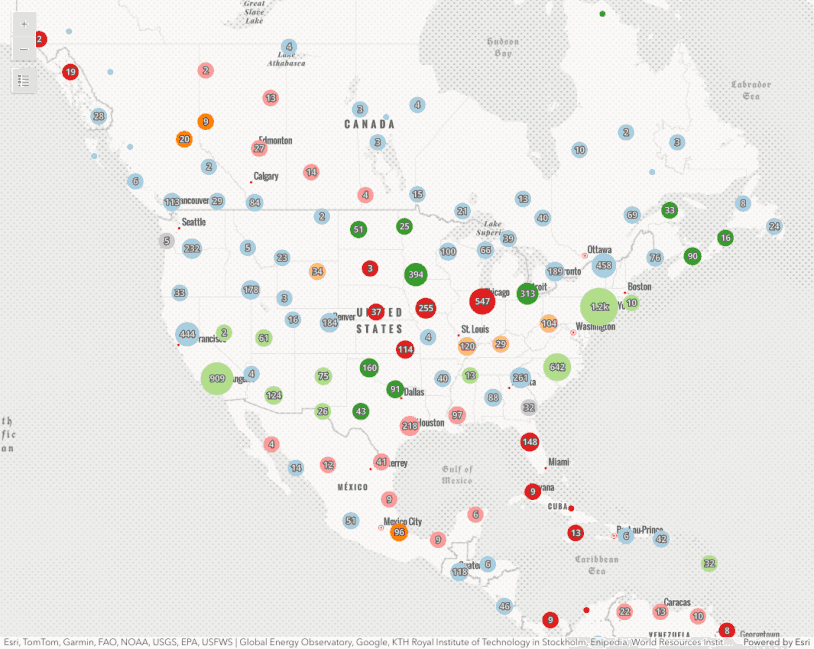
Clustering - query clusters
Clustering - query clusters
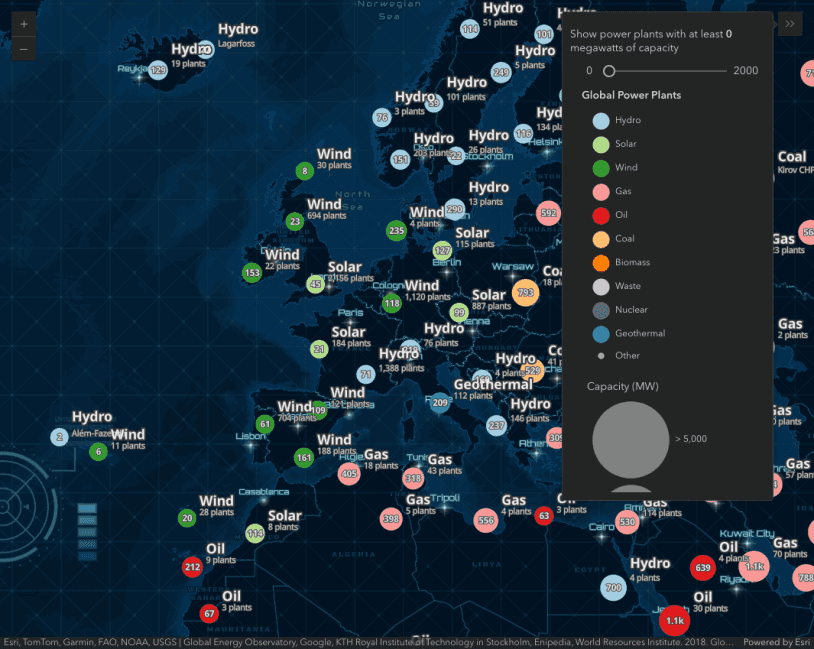
Clustering - advanced configuration
Clustering - advanced configuration
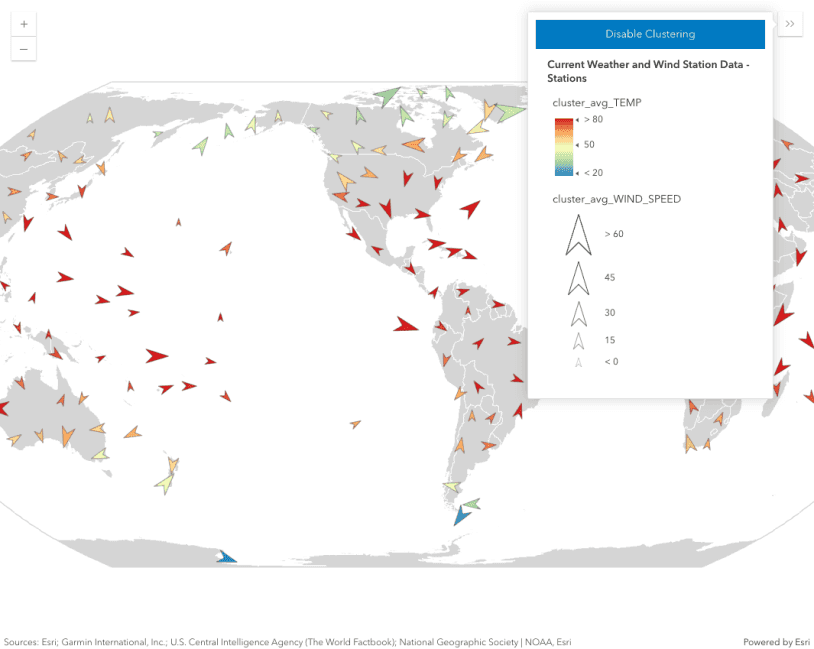
Point clustering with visual variables
Point clustering with visual variables
FeatureReductionCluster
Read the API Reference for more information.
API support
The following table describes the geometry and view types that are suited well for each visualization technique.
2D | 3D | Points | Lines | Polygons | Mesh | Client-side | Server-side | |
---|---|---|---|---|---|---|---|---|
Clustering | ||||||||
Binning | ||||||||
Heatmap | ||||||||
Opacity | ||||||||
Bloom | ||||||||
Aggregation | ||||||||
Thinning | 1 | 1 | 1 | 2 | 3 | |||
Visible scale range |
- 1. Feature reduction selection not supported
- 2. Only by feature reduction selection
- 3. Only by scale-driven filter